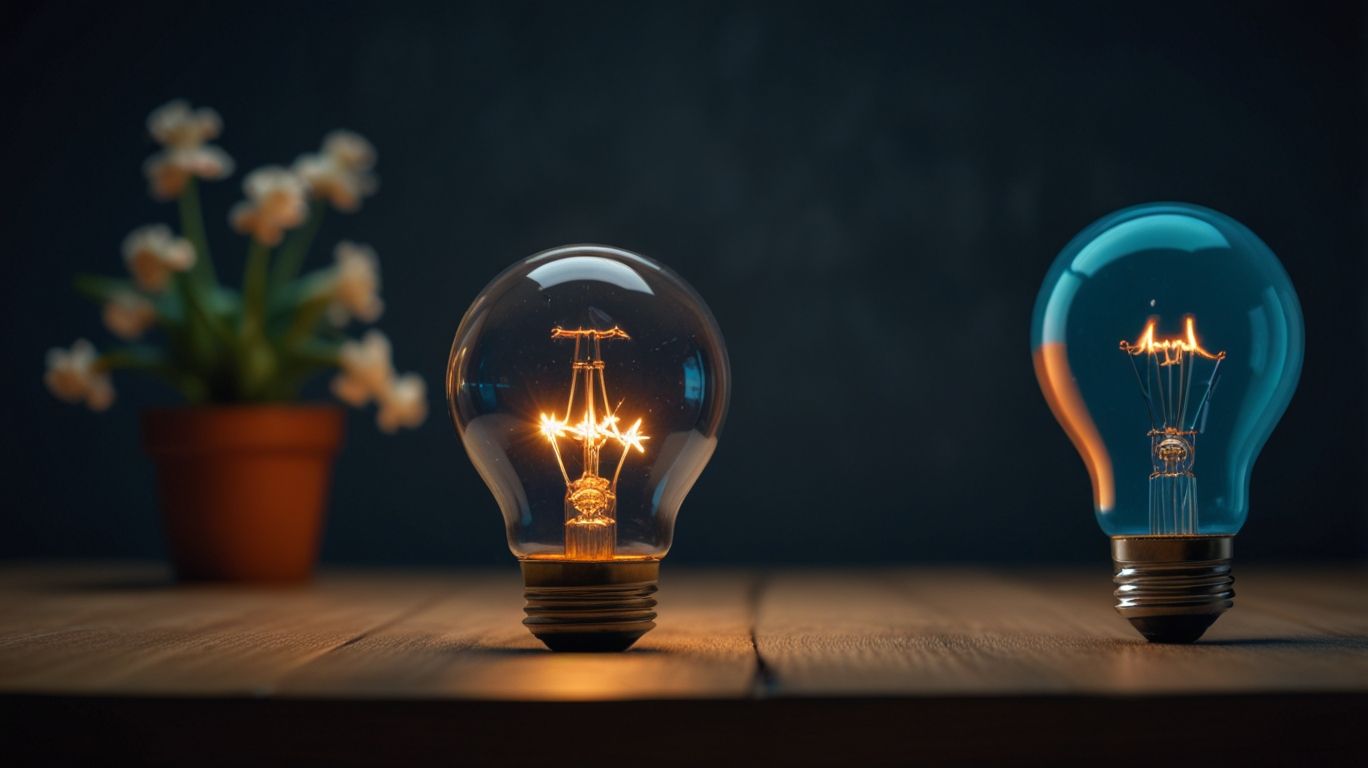
Understanding the Difference Between LLM and Reasoning Models
Introduction to LLMs (Large Language Models)
Large Language Models (LLMs) represent a sophisticated class of artificial intelligence designed to understand, generate, and manipulate human language at scale. Built primarily on transformer architecture, these models leverage vast amounts of textual data to learn the nuances of language, including grammar, context, and semantics. One of the core principles behind LLMs is their capacity to predict the subsequent word in a sequence, which lays the groundwork for more complex language tasks. This predictive capability is achieved through training on diverse datasets that encompass books, articles, and other text forms, allowing LLMs to absorb a wide range of linguistic patterns.
The training process for LLMs involves two key phases: pre-training and fine-tuning. During pre-training, the models ingest and analyze large datasets, learning to identify patterns and relationships within the text. This stage equips them with a general understanding of language. Fine-tuning follows, where the model is adjusted using specific datasets tailored to particular tasks, enhancing its performance in targeted applications, such as translation or sentiment analysis. Notably, models like OpenAI’s GPT-3 and Google’s BERT exemplify the advancements in this field. GPT-3 leverages its extensive training to produce human-like text responses across varied prompts, while BERT specializes in understanding the context of words within sentences.
Data inputs for LLMs are predominantly unstructured text, which allows for a broad range of applications, from chatbot development to content creation. As these models evolve, their ability to understand context and generate coherent, contextually relevant outputs continues to improve, leading to new possibilities in communication technology. Understanding the architecture and functioning of LLMs is crucial for appreciating their significance in today’s AI-driven landscape.
What are Reasoning Models?
Reasoning models are computational frameworks designed to emulate human reasoning processes, such as problem-solving, logical deduction, and abstract thinking. These models serve as essential tools in the fields of artificial intelligence (AI) and cognitive science, advocating the development of systems that can interpret, analyze, and draw conclusions from information in a manner similar to human cognitive function.
At their core, reasoning models utilize a structured approach to represent knowledge and infer new information. They can be categorized into several types, each relying on distinct methodologies. One notable category is symbolic reasoning, where knowledge is represented using symbols that correspond to real-world entities or concepts. This approach is particularly effective in domains where clear, logical representation is essential, such as mathematics and formal logic.
Another prominent type of reasoning model is rule-based systems. These systems operate on a set of predefined rules, harnessing a knowledge base that consists of facts and inference rules. When supplied with specific inputs, rule-based systems apply logical reasoning to derive outcomes or solutions based on the established rules. This method is widely used in expert systems, where the aim is to simulate the expertise of human specialists in fields like medical diagnosis or financial forecasting.
Reasoning models have numerous applications across various domains, including natural language processing, automated theorem proving, and even complex decision-making scenarios in business environments. Their ability to simulate human-like reasoning capabilities makes them invaluable for enhancing AI systems, allowing machines to perform tasks that traditionally necessitated human intelligence.
In summary, reasoning models encapsulate a significant segment of cognitive modeling in AI, facilitating a deeper understanding and advancement in how machines can replicate human reasoning capabilities effectively.
Key Differences Between LLMs and Reasoning Models
Large Language Models (LLMs) and reasoning models represent two distinct paradigms in the realm of artificial intelligence, each with unique strengths and weaknesses. LLMs, such as OpenAI’s GPT series, primarily excel in processing and generating natural language. They utilize vast datasets to learn patterns, grammar, and nuances in various languages, enabling them to produce contextually relevant and coherent text. However, their reasoning capabilities may be limited, especially in scenarios requiring logical deduction or problem-solving.
On the other hand, reasoning models are designed specifically for tasks that involve logical reasoning and structured problem-solving. These models often rely on rule-based systems or formal logic frameworks, enabling them to tackle problems involving deduction, induction, and abduction effectively. For example, reasoning models excel in applications such as mathematical theorem proving and complex decision-making scenarios, where the logical flow of information is crucial.
The way these models handle data also differs significantly. LLMs take a more generalized approach by training on extensive datasets to capture linguistic diversity, which enables them to generate language in a conversational manner. In contrast, reasoning models often work with narrowly defined datasets where the relationships between inputs and outputs are explicitly established, allowing them to perform more accurately in specific domains.
Moreover, the typical use cases for LLMs and reasoning models highlight their differences. LLMs are commonly employed for content generation, chatbots, and language translation, where natural language processing is essential. Conversely, reasoning models find their applications in fields requiring complex reasoning, such as legal document analysis, scientific research, and automated reasoning tasks.
By recognizing these key differences, practitioners can better choose the appropriate model for their specific AI applications, ensuring that they harness the strengths of each approach effectively.
Future of LLMs and Reasoning Models in AI Development
The ongoing evolution of artificial intelligence is significantly shaped by the advancements in large language models (LLMs) and reasoning models. As industries increasingly adopt AI solutions, the integration of both types of models has become a focal point of development efforts. Currently, there is a discernible trend towards harmonizing the strengths of LLMs with those of reasoning models to create composite systems that leverage their respective capabilities. LLMs excel in natural language processing, enabling them to understand and generate human-like text, while reasoning models contribute logical inference and problem-solving abilities.
With advancements in machine learning algorithms and computational power, the future promises even more sophisticated iterations of these models. For instance, the incorporation of reasoning capabilities into LLMs may lead to systems that not only generate coherent text but also provide sound reasoning and evidential support for their responses. This synergy would significantly enhance user interaction, making AI tools more reliable and efficient in practical applications, from customer service to complex decision-making tasks.
Moreover, as we move forward, ethical considerations and usability will play pivotal roles in shaping the future landscape of these technologies. The deployment of LLMs and reasoning models must involve stringent guidelines to ensure that AI systems are used responsibly. They must guard against biases and ensure transparency in operations, ultimately fostering user trust. Industries, especially those heavily reliant on data analysis, such as finance, healthcare, and education, stand to benefit greatly from these advancements, reinforcing the need for continued research and ethical frameworks surrounding AI technologies.
In conclusion, the momentum towards integrating LLMs and reasoning models signals a promising future for AI development, where enhanced capabilities will meet ethical considerations, driving innovation across various sectors.